Detección de fuerza y posición para los movimientos de flexión-extensión de codo a partir de señales de EMG
Force and position detection for elbow flexion and extension movements from EMG signals
Barra lateral del artículo
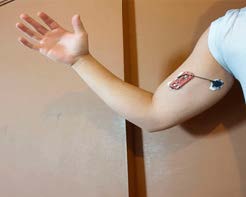
Términos de la licencia (VER)

Esta obra está bajo una licencia internacional Creative Commons Atribución-NoComercial-SinDerivadas 4.0.
Declaración del copyright
Los autores ceden en exclusiva a la Universidad EIA, con facultad de cesión a terceros, todos los derechos de explotación que deriven de los trabajos que sean aceptados para su publicación en la Revista EIA, así como en cualquier producto derivados de la misma y, en particular, los de reproducción, distribución, comunicación pública (incluida la puesta a disposición interactiva) y transformación (incluidas la adaptación, la modificación y, en su caso, la traducción), para todas las modalidades de explotación (a título enunciativo y no limitativo: en formato papel, electrónico, on-line, soporte informático o audiovisual, así como en cualquier otro formato, incluso con finalidad promocional o publicitaria y/o para la realización de productos derivados), para un ámbito territorial mundial y para toda la duración legal de los derechos prevista en el vigente texto difundido de la Ley de Propiedad Intelectual. Esta cesión la realizarán los autores sin derecho a ningún tipo de remuneración o indemnización.
La autorización conferida a la Revista EIA estará vigente a partir de la fecha en que se incluye en el volumen y número respectivo en el Sistema Open Journal Systems de la Revista EIA, así como en las diferentes bases e índices de datos en que se encuentra indexada la publicación.
Todos los contenidos de la Revista EIA, están publicados bajo la Licencia Creative Commons Atribución-NoComercial-NoDerivativa 4.0 Internacional
Licencia
Esta obra está bajo una Licencia Creative Commons Atribución-NoComercial-NoDerivativa 4.0 Internacional
Contenido principal del artículo
Resumen
Objetivo: Evaluar fuerza y posición angular a partir del análisis de señales electromiográficas en bíceps y tríceps durante la realización de movimientos de flexión-extensión de codo, comparando los algoritmos V − Order, WaveLength, Mean Absolute Value, Wavelength y un factor denominado Valor Q1.
Materiales y métodos: Se realizó un experimento con 15 voluntarios con cargas de 0, 0.5, y 1 kg. Para determinar la fuerza, se extraen características de la señal teniendo en cuenta los algoritmos mencionados en el objetivo, y a partir de estos valores se halla la posición angular mediante el uso de un filtro pasabajos. Para validar los algoritmos, se utilizó un sensor comercial NOTCH para la medición de posición angular y se evaluó el coeficiente de correlación comparando con los resultados del algoritmo que presenta mejor comportamiento.
Resultados: Al evaluar las señales adquiridas, la señal del bíceps presenta mayor amplitud respecto al tríceps. Adicionalmente, el algoritmo Q1 presenta menores niveles de ruido por lo que se utiliza para determinar la posición angular. Los resultados muestran que el método Valor Q1 permite la estimación de la señal de posición angular, con un coeficiente de correlación promedio de 72,3% para 1 kg y 60,9% para 0 kg.
Conclusiones: La medición de fuerza y posición angular permite el desarrollo de sistemas de control para dispositivos biomecatrónicos destinados al apoyo de procesos de rehabilitación y evaluación de la evolución de un paciente. Los resultados fueron mejores con pesos mayores, debido a que esto implica mayor activación muscular.
Descargas
Detalles del artículo
Referencias (VER)
A. G. Noughaby and G. R. Vossoughi, “The Control of an Exoskeleton and The Reduction of Interaction Force Using Human Intent Detection by EMG Signals and Torque Estimation,” 2018 6th RSI International Conference on Robotics and Mechatronics (IcRoM), 2018, pp. 536-541, doi: 10.1109/ICRoM.2018.8657584.
A. Zonnino and F. Sergi, “Model-Based Estimation of Individual Muscle Force Based on Measurements of Muscle Activity in Forearm Muscles During Isometric Tasks,” in IEEE Transactions on Biomedical Engineering, vol. 67, no. 1, pp. 134-145, Jan. 2020, doi: 10.1109/TBME.2019.2909171.
Bingke Zhang and Shiyou Zhang, “The estimation of grasping force based on the feature extracted from EMG signals,” 2016 IEEE Advanced Information Management, Communicates, Electronic and Automation Control Conference (IMCEC), 2016, pp. 1477-1480, doi: 10.1109/IMCEC.2016.7867461.
Bueno, A. J., & Porqueres, I. M. (2007). MANUAL DE PRUEBAS DIAGNÓSTICAS. Traumatología y ortopedia (Vol. 85). Editorial Paidotribo. ISBN: 978-84-9910-129-3
C. Liang and T. Hsiao, “Admittance Control of Powered Exoskeletons Based on Joint Torque Estimation,” in IEEE Access, vol. 8, pp. 94404-94414, 2020, doi: 10.1109/ACCESS.2020.2995372.
Cristancho, W. (2012). Fisioterapia en la UCI: Teoría, experiencia y evidencia. Bogotá, Colombia: Manual Moderno. ISBN: 9789589446676
E. P. Doheny, C. Goulding, M. W. Flood, L. Mcmanus and M. M. Lowery, “Feature-Based Evaluation of a Wearable Surface EMG Sensor Against Laboratory Standard EMG During Force-Varying and Fatiguing Contractions,” in IEEE Sensors Journal, vol. 20, no. 5, pp. 2757-2765, 1 March1, 2020, doi: 10.1109/JSEN.2019.2953354.
G. Hajian, A. Etemad and E. Morin, “An Investigation of Dimensionality Reduction Techniques for EMG-based Force Estimation,” 2019 41st Annual International Conference of the IEEE Engineering in Medicine and Biology Society (EMBC), 2019, pp. 698-701, doi: 10.1109/EMBC.2019.8856293.
I. J. R. Martínez, A. Mannini, F. Clemente and C. Cipriani, “Online Grasp Force Estimation from the Transient EMG,” in IEEE Transactions on Neural Systems and Rehabilitation Engineering, vol. 28, no. 10, pp. 2333-2341, Oct. 2020, doi: 10.1109/TNSRE.2020.3022587.
J. B. Ullauri, L. Peternel, B. Ugurlu, Y. Yamada and J. Morimoto, “On the EMG-based torque estimation for humans coupled with a force-controlled elbow exoskeleton,” 2015 International Conference on Advanced Robotics (ICAR), 2015, pp. 302-307, doi: 10.1109/ICAR.2015.7251472.
J. Hashemi, E. Morin, P. Mousavi and K. Hashtrudi-Zaad, “Enhanced Dynamic EMG-Force Estimation Through Calibration and PCI Modeling,” in IEEE Transactions on Neural Systems and Rehabilitation Engineering, vol. 23, no. 1, pp. 41-50, Jan. 2015, doi: 10.1109/TNSRE.2014.2325713.
Josten, C., y Lill, H. (2006). Lesiones del codo: biomecánica, diagnóstico, tratamiento. Amolca. ISBN: 9789806574106
M. Jabbari, R. N. Khushaba and K. Nazarpour, “EMG-Based Hand Gesture Classification with Long Short-Term Memory Deep Recurrent Neural Networks,” 2020 42nd Annual International Conference of the IEEE Engineering in Medicine & Biology Society (EMBC), 2020, pp. 3302-3305, doi: 10.1109/EMBC44109.2020.9175279.
M. Kasuya, M. Seki, K. Kawamura, Y. Kobayashi, M. G. Fujie and H. Yokoi, “Robust grip force estimation under electric feedback using muscle stiffness and electromyography for powered prosthetic hand,” 2013 IEEE International Conference on Robotics and Automation, 2013, pp. 93-98, doi: 10.1109/ICRA.2013.6630561.
Márquez García, F. J. (2013). Evaluación de la fuerza relativa de las extremidades superiores con la plataforma de Bosco.
Nesbit, S. M., Elzinga, M., Herchenroder, C., & Serrano, M. (2006). The effects of racket inertia tensor on elbow loadings and racket behavior for central and eccentric impacts. Journal of sports science & medicine, 5(2), 304.
O. Bida, D. Rancourt and E. A. Clancy, “Electromyogram (EMG) amplitude estimation and joint torque model performance,” Proceedings of the IEEE 31st Annual Northeast Bioengineering Conference, 2005., 2005, pp. 229-230, doi: 10.1109/NEBC.2005.1432004.
Pasnoor, M., Dimachkie, M. M., Farmakidis, C., y Barohn, R. J. (2019). Disorders of the neuromuscular junction: Myasthenia gravis. En R. Mitra (Ed.), Principles of rehabilitation medicine. New York, NY: McGraw-Hill Education.
Wang, H., Rajotte, K. J., Wang, H., Dai, C., Zhu, Z., Bhuiyan, M., ... & Clancy, E. A. (2019). Optimal Estimation of EMG Standard Deviation (EMG $sigma $) in Additive Measurement Noise: Model-Based Derivations and Their Implications. IEEE Transactions on Neural Systems and Rehabilitation Engineering, 27(12), 2328-2335.
X. Jiang, B. Bardizbanian, C. Dai, W. Chen and E. A. Clancy, “Data Management for Transfer Learning Approaches to Elbow EMG-Torque Modeling,” in IEEE Transactions on Biomedical Engineering, vol. 68, no. 8, pp. 2592-2601, Aug. 2021, doi: 10.1109/TBME.2021.3069961.
Y. Chen, C. Dai and W. Chen, “Cross-Comparison of EMG-to-Force Methods for Multi-DoF Finger Force Prediction Using One-DoF Training,” in IEEE Access, vol. 8, pp. 13958-13968, 2020, doi: 10.1109/ACCESS.2020.2966007.
Y. Zhuang, Y. Leng, J. Zhou, R. Song, L. Li and S. W. Su, “Voluntary Control of an Ankle Joint Exoskeleton by Able-Bodied Individuals and Stroke Survivors Using EMG-Based Admittance Control Scheme,” in IEEE Transactions on Biomedical Engineering, vol. 68, no. 2, pp. 695-705, Feb. 2021, doi: 10.1109/TBME.2020.3012296.