Comparison of different spatial filters for identification of the gray matter in brain imaging
Comparación de diferentes filtros espaciales para la identificación de la materia gris en imágenes cerebrales
Barra lateral del artículo
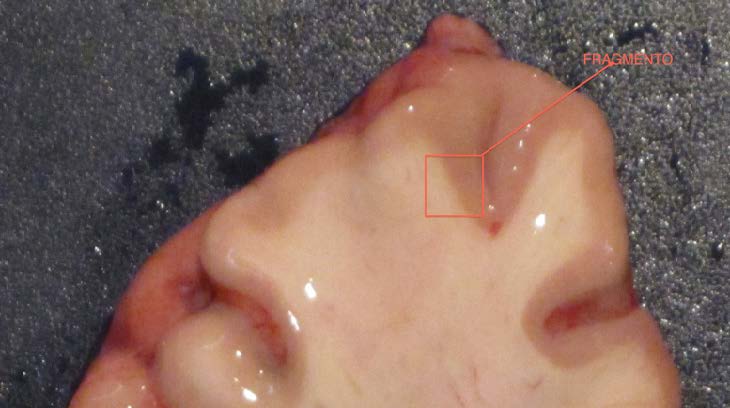
Términos de la licencia (VER)

Esta obra está bajo una licencia internacional Creative Commons Atribución-NoComercial-SinDerivadas 4.0.
Declaración del copyright
Los autores ceden en exclusiva a la Universidad EIA, con facultad de cesión a terceros, todos los derechos de explotación que deriven de los trabajos que sean aceptados para su publicación en la Revista EIA, así como en cualquier producto derivados de la misma y, en particular, los de reproducción, distribución, comunicación pública (incluida la puesta a disposición interactiva) y transformación (incluidas la adaptación, la modificación y, en su caso, la traducción), para todas las modalidades de explotación (a título enunciativo y no limitativo: en formato papel, electrónico, on-line, soporte informático o audiovisual, así como en cualquier otro formato, incluso con finalidad promocional o publicitaria y/o para la realización de productos derivados), para un ámbito territorial mundial y para toda la duración legal de los derechos prevista en el vigente texto difundido de la Ley de Propiedad Intelectual. Esta cesión la realizarán los autores sin derecho a ningún tipo de remuneración o indemnización.
La autorización conferida a la Revista EIA estará vigente a partir de la fecha en que se incluye en el volumen y número respectivo en el Sistema Open Journal Systems de la Revista EIA, así como en las diferentes bases e índices de datos en que se encuentra indexada la publicación.
Todos los contenidos de la Revista EIA, están publicados bajo la Licencia Creative Commons Atribución-NoComercial-NoDerivativa 4.0 Internacional
Licencia
Esta obra está bajo una Licencia Creative Commons Atribución-NoComercial-NoDerivativa 4.0 Internacional
Contenido principal del artículo
Resumen
Objetivo: comparar diferentes filtros espaciales para la identificación de la materia gris en imágenes cerebrales, con el fin de mejorar el contraste en las imágenes y facilitar su interpretación en diagnósticos médicos. Materiales: se seleccionaron diez filtros espaciales que fueron implementados y evaluados mediante una interfaz gráfica de usuario (GUI) en Matlab. Los filtros incluyen desde conversiones a escala de grises hasta filtros más complejos como el gaussiano y el de enfoque. La eficacia de cada filtro se midió a través del coeficiente de correlación entre las curvas de intensidad de píxeles generadas por los filtros y una curva modelo ideal. Resultados: el filtro espacial no lineal de mediana fue el que mostró el coeficiente de correlación más alto (0.8974), indicando que se aproxima mejor a la curva modelo ideal para la diferenciación entre materia blanca y gris. En contraste, el filtro de relieve (emboss) mostró el coeficiente más bajo (0.6972), lo que sugiere que no es adecuado para la identificación de tejidos. Conclusiones: el filtro de mediana demostró ser el más efectivo para la identificación de la materia gris en las imágenes cerebrales. La investigación sugiere que combinaciones de diferentes filtros pueden mejorar aún más los resultados, y que la GUI desarrollada permite explorar exhaustivamente estas combinaciones para optimizar la filtración y análisis de imágenes médicas.
Descargas
Detalles del artículo
Referencias (VER)
Andersen, L. & Dalal, S. (2022). Corrigendum to the cerebellar clock: Predicting and timing somatosensory touch. NeuroImage, 238, https://doi.org/10.1016/j.neuroimage.2022.119238.
Antl, I. (2020). Programa de manipulación de imágenes de GNU. Informe técnico. Equipo de documentación de GIMP, España.
Bao, H., Zhou, H., Li, J., Pei, H., Tian, J., Yang, L., & Miao, X. (2022). Toward memristive in-memory computing: Principles and applications. Frontiers of Optoelectronics, 15(1), https://doi.org/10.1007/s12200-022-00025-4.
Chauhan, R., Shah, T., Shah, D., Gohil, T., Oza, A., Jajal, B. & Saxena, K. (2023). An overview of image processing for dental diagnosis. Innovation and Emerging Technologies, 10(2330001), https://doi.org/10.1142/S2737599423300015.
Chen, Z., Pawar, K., Ekanayake, M., Pain, C., Zhong, S. & Egan, G. (2023). Deep learning for image enhancement and correction in magnetic resonance imaging—state-of-the-art and challenges. Journal of Digital Imaging, 36(1), 204-230, https://doi.org/10.1007/s10278-022-00721-9.
Debevec, P., Taylor, C. & Malik, J. (2023). Modeling and rendering architecture from photographs: A hybrid geometry-and image-based approach. In Seminal Graphics Papers: Pushing the Boundaries, 2, 465-474, https://doi.org/10.1145/237170.237191.
Escalante, B. (2006). Procesamiento digital de imágenes. Documento técnico. Facultad de Ingeniería. Universidad Nacional Autónoma de México.
Goceri, E. (2023). Medical image data augmentation: Techniques, comparisons and interpretations. Artificial Intelligence Review, 56(11), 12561-12605, https://doi.org/10.1007/s10462-023-10453-z.
González, R. & Woods R. (2018). Digital image processing. Fourth edition. Pearson Prentice Hall, Pearson Education International. New York, USA.
Govindhan, A., Sunitha, K. A. & Kandhasamy, S. (2023). A custom manipulator for dental implantation through model-based design. Intelligent Automation and Soft Computing, 35(1), 351-365, https://doi.org/10.32604/iasc.2023.026361.
Hamid, M. & Khan, N. (2020). Investigation and classification of MRI brain tumors using feature extraction technique.
Journal of Medical and Biological Engineering, 40(2), 307-317, https://doi.org/10.1007/s40846-020-00510-1.
Isufi, E., Gama, F., Shuman, D. & Segarra, S. (2024). Graph filters for signal processing and machine learning on graphs. IEEE Transactions on Signal Processing, (99), 1-32, https://doi.org/ 10.1109/TSP.2024.3349788.
Jawad, H., Hasan, H., Abdulqader, A., Kovalevskaya, L. & Kozubtsov, I. (2024). The detection of brain tumors user interface for MATLAB. In 2024 35th Conference of Open Innovations Association (IEEE FRUCT), 319-327, https://doi.org/10.23919/FRUCT61870.2024.10516406.
Kaur, R. & Devendran, V. (2023). Image matching techniques: A review. Lecture Notes in Networks and Systems, 785-795, https://doi.org/10.1007/978-981-19-0098-3_74.
Khudhair, Z., Khdiar, A., El Abbadi, N., Mohamed, F., Saba, T., Alamri, F. & Rehman, A. (2023). Color to grayscale image conversion based on singular value decomposition. IEEE Access, 11, 54629-54638, https://doi.org/10.1109/ACCESS.2023.3279734.
Lavanya, N. & Nagasundaram, S. (2023). Improving brain tumor MRI images with pre-processing techniques for noise removal. In 2023 International Conference on Sustainable Communication Networks and Application (IEEE ICSCNA), 1530-1538, https://doi.org/10.1109/ICSCNA58489.2023.10370349.
Lepcha, D., Goyal, B., Dogra, A., Sharma, K. & Gupta, D. (2023). A deep journey into image enhancement: A survey of current and emerging trends. Information Fusion, 93, 36-76, https://doi.org/10.1016/j.inffus.2022.12.012.
Lukin, V., Proskura, G. & Vasilyeva, I. (2019). Improvement of multichannel image classification by combining elementary classifiers. 2019 IEEE International Scientific-Practical Conference: Problems of Infocommunications Science and Technology, 660-664. https://doi.org/10.1109/PICST47496.2019.9061380.
Mall, P., Singh, P., Srivastav, S., Narayan, V., Paprzycki, M., Jaworska, T. & Ganzha, M. (2023). A comprehensive review of deep neural networks for medical image processing: Recent developments and future opportunities. Healthcare Analytics, 4(100216), https://doi.org/10.1016/j.health.2023.100216.
Mirza, M., Siddiq, A. & Khan, I. (2023). A comparative study of medical image enhancement algorithms and quality assessment metrics on COVID-19 CT images. Signal, Image and Video Processing, 17(4), 915-924, https://doi.org/10.1007/s11760-022-02214-2.
Núñez, M. (2008). Funciones del tecnólogo en el equipo multidisciplinario de medicina nuclear e interacción con el paciente. Informe técnico. Escuela Universitaria de Tecnología Médica, Montevideo, Uruguay.
Pannekoucke, O., Raynaud, L. & Farge, M. (2014). A wavelet-based filtering of ensemble background-error variances. Quarterly Journal of the Royal Meteorological Society, 140(678), 316-327, https://doi.org/10.1002/qj.2133.
Rasheed, M., Iqbal, M., Jaffar, A., Ashraf, M., Almarhabi, K., Alghamdi, A. & Bahaddad, A. (2023). Recognizing brain tumors using adaptive noise filtering and statistical features. Diagnostics, 13(8), 1451, https://doi.org/10.3390/diagnostics13081451.
Stanković, L. & Mandic, D. (2023). Convolutional neural networks demystified: A matched filtering perspective-based tutorial. IEEE Transactions on Systems, Man, and Cybernetics: Systems, 53(6), 3614-3628, https://doi.org/10.1109/TSMC.2022.3228597.
Quqa, S., Lasri, O. & Landi, L. (2023). Bridge monitoring using vehicle-induced vibration. Lecture Notes in Civil Engineering, vol. 254 LNCE, 59-67, https://doi.org/10.1007/978-3-031-07258-1_7.